AI and Epistemic Risk: A Coming Crisis?
Justin Hendrix / Jun 10, 2024Audio of this conversation is available via your favorite podcast service.
What are the risks to democracy as AI is incorporated more and more into the systems and platforms we use to find and share information and engage in communication?
For this episode, I spoke with Elise Silva, a postdoctoral associate at the University of Pittsburgh Cyber Institute for Law, Policy, and Security, and John Wihbey, an associate professor at Northeastern University in the College of Arts, Media and Design. Silva is the author of a recent piece in Tech Policy Press titled "AI-Powered Search and the Rise of Google’s 'Concierge Wikipedia.'” Wihbey is the author a paper published last month titled "AI and Epistemic Risk for Democracy: A Coming Crisis of Public Knowledge?"
What follows is a lightly edited transcript of the discussion.
Justin Hendrix:
Good morning, I'm Justin Hendrix, Editor of Tech Policy Press, a non-profit media venture intended to promote new ideas, debate and discussion, at the intersection of technology and democracy.
Like it or not, some of the biggest technology firms seem intent on lacing artificial intelligence into every platform in which internet users search for information and communicate with one another. What impact will that have on democracy? Will chatbots and other purportedly intelligent agents play a significant role in mediating the information we consume and have some significant impact on the beliefs we hold? What is the epistemic risk to democracy from artificial intelligence, and how do we develop AI systems support, rather than undermine the principles of an informed and engaged citizenry?
Today, I'm joined by two scholars who are thinking and writing about this question.
Elise Silva:
My name's Elise Silva and I'm a postdoctoral associate at the University of Pittsburgh's Cyber Institute for Law Policy and Security. I have a PhD from the University of Pittsburgh and a Masters of library and information science. And my current research interests revolve around information ecosystems. I work in areas like misinformation and disinformation, how people find, evaluate and use information, and generally, the sociotechnical realities of information ecosystems and how tech and information evolve together.
John Wihbey:
So my name is John Wihbey, I'm an Associate Professor at Northeastern University in the College of Arts Media and Design, and my work and teaching focus a lot on news media and social media and their intersection. And I'm increasingly studying this concept of the information environment, so taking the media ecosystem and looking at it at multiple levels. The latest thing I'm doing is helping to found something called the Internet Democracy Initiative with David Lazar, which is in part a computational social science effort, but also an attempt to look at the information environment critically in multiple dimensions.
Justin Hendrix:
I'm excited to have both of you on this podcast and these topics are core to what we try to discuss here week in, week out. And I got in touch with you, John, after the publication of a paper that came out just beginning of May on AI and Epistemic Risk for Democracy, a Coming Crisis of Public Knowledge. And then Elise came to us with a piece, Protect Policy Press, in the midst of Google's launch of AI-powered search, which she called AI-powered Search and the Rise of Google's Concierge Wikipedia, which raised some similar kinds of concerns, but of course pegged to what's going on at the moment with Google.
So I thought I might start actually with you, Elise, just on the kind of news of the day, what you've been observing around the rollout of AI overviews. Since you published this piece, of course Google has stepped back a bit from the launch of this, but it seems clear that generative AI and large language models and all of the various accoutrements that come along with those systems are going to be embedded in our search environment no matter what happens to this current iteration of AI overviews. What are you thinking about at the moment, in terms of this particular episode?
Elise Silva:
Yeah, I have been waiting for this announcement for quite some time, really, with some anticipation. I expected there would be a lot of excitement and some angst. I have seen so much more angst than I thought I would, and a lot of that I think, revolves around two things. The first is the quality of the information that the LLM, the technology is providing to the user. And then the second is some concern about what this is going to do for online buying, commerce and where internet traffic is going to be a pointed, with Google essentially being an advertising platform, people are worried about these things.
I am still, tend to be a little bit more interested in how users themselves, how their searching practices are going to change. And so a lot of that's just being intuited by me at this point, knowing what I already know about how people use these interfaces. But I am looking forward to longer term studies about how people are interacting with these information and objects, what this synthesis is looking like for them, how that's affecting their ability to critically think about information, what it means in terms of citation politics and who is getting recognized. So these are some central questions that I have that I think longer term ones.
Justin Hendrix:
Of course, that's where I see the parallel to John's interests in this paper. You ask what you call a central research question, what are the dangers to public knowledge and the concept of an informed citizenry in a democracy that is increasingly suffused with and mediated by advanced AI technologies? John, what are the kind of, I don't know, what's the taxonomy around these questions that you're trying to develop or the conceptual framework or rubric that you're trying to look at this question through?
John Wihbey:
The thing that really catalyzed my thinking was just this obsession with existential risk and x-risk as it's called, and I thought killer robots and their undermining of human agency, I certainly see that as a potential, obviously a huge potential problem. I thought there's probably a lot of middle tier problems that are going to start to emerge as these technologies begin to mediate the entire information environment.
And in terms of a taxonomy or an analytical framework, I started to try to divide the information and environment as mediated by AI into, they just to be the categories that I have some background in, but there are many more, and these were journalism, social media, and content moderation specifically, and then polling and public opinion. I glanced at search, I wrote the paper before the Google AI overview announcement was made, but I was also thinking about search. I was thinking about the ways in which all these domains will change or be changed or are changing in some cases. Obviously, neural nets and deep learning are being used in some of these domains already, especially with social media.
And what I'm trying to do is think broadly about, what does it mean collectively if all of these are changed together? And what does it mean for human experience, democratic deliberation and what could be the potential risks within it? And the central risk I see is that the AI models are trained on the human past and much of what is important I think in democratic societies in particular is the emergent phenomena that are not yet predictable through models, even if they're well-trained and there's lots of feedback being inputted.
Justin Hendrix:
And Elise, this is another topic you've written about specifically for Tech Policy Press and also in your research through the lens of gender and looking at the way that the internet of the past, which of course is the basis for the models and the tools that being rolled out by these firms today, is a largely male construction.
Elise Silva:
Yeah, absolutely. In previous work, and this was really hard actually for us to estimate, but we estimated around 26% potentially of earlier versions of ChatGPT models were trained on data that was written by women, language from women, writing from women. And so not only is this a past looking thing, but that past has a very particular flavor because of who has been represented in that past and who has not been represented in that past. So I really appreciate that thought about evolution and giving up a space to get better, to provide better data, to provide more representative data that just looking at the past in this enormous amount of data that's making these systems work, but making them work really unevenly across different representative spheres.
I'll also say that something else I've been thinking of and reading about is model collapse and that this isn't good for people necessarily or democracy, but it's also not necessarily good for the models themselves if they're just training on the same data that they're putting out in order just to get bigger and better. So, I think that these risks are for people, these risks are for particularly marginalized communities, and these risks are for, should be of interest to those developing the tech.
Justin Hendrix:
Of course, those risks extend beyond just gender inequities, race, language, geography, connectivity as a kind of just basis for whether people are on the internet or not or in any way represented in the vast amounts of information that's been hoovered up here.
John, you accept that reinforcement learning techniques, other types of add-ons and fixes that AI companies are imagining might overcome some of these deficits. But are you optimistic that's going to be the case in the near term and if not, what does that mean?
John Wihbey:
Reinforcement learning through human feedback, which is the sort of term of art in the industry, is being done at a vast scale and I'm not a technical expert at all, I only know what I read and what colleagues tell me, but it does seem that you can improve the models quite a bit along some of these lines, in fact, along some of the biases that are embedded. But it's not clear to me given the breadth of human communication, experience and knowledge that you can cover all of the bases all of the time and that there's always going to be slippage, there are always going to be gaps, and I really worry about these feedback loops where AI condition us to believe X and then we believe X and then we behave and communicate in such a way that's conditioned by that and then that is fed back into the model.
So I think it's a broad, maybe even a philosophical point. I worry about bias being locked in, but I also worry about human tacit knowledge, gut feelings, emotion. A lot of the things that are just fundamentally emergent dynamics of human experience and learning, just being crowded out, even as the models get better and try to mediate in a more flexible way that's attentive to emerging dynamics. I think in the area of knowledge, I think there are a lot of dangers that we haven't considered and also even as the models might be able to bring in more human input, so that the sort of bit rate of preference communication could be increased vastly, but how that's then filtered through a model of what's important and then condensed and summarized I think is going to be terribly important.
Justin Hendrix:
Elise, what about from an information science perspective? Do you think that we're in the near term going to get past some of these issues? People have proposed all sorts of ways of solving some of these problems from synthetic data to trying to hoover up more and more information from across the world. Do you think it's addressable?
Elise Silva:
I don't know that I am the expert to say if it is or isn't. What I do know is that I am in touch with a lot of very smart and very well-meaning machine learning experts who are working in an ethical AI space and genuinely want it to work. And they're doing things like including public participation as part of creating models, they're doing wider user testing, they're thinking I think more expansively about these things. And so just a shout-out to all of the people in that space that are doing that work.
I will say that just in terms of we were talking about training data and then mitigation as an answer to this is just at the end, right? It's at the end. It doesn't necessarily deal with the root cause. The root cause is that we just don't have the expansive amount of data that we need. We have a lot of it, but the quality of that data might not be what we need. And so I'm interested in a visionary future of what it would look like to not retrofit or mitigate from the end, but what it looks like to create models with better data from the beginning.
Justin Hendrix:
Talking specifically about journalism and John, you spend quite a lot of time in your paper focusing on different critiques of use of artificial intelligence in journalism. One I found in particular was just the thought that more and more automation means less and less connection between journalists and the people they cover, the extent to which that's a grave concern. You put forward this hypothetical, which I imagine is literally the startup plan that we see in probably many entrepreneurs' minds at the moment, which is, somebody with an idea to automate news gathering, wanders into a news desert and sees what they can do. Explain this hypothetical, why you think it's problematic.
John Wihbey:
Sure, and the term news desert, Penny Abernathy was a researcher, coined this term, but it's this idea that there are micro or local or even regional media environments or information environments across the United States and obviously across the world, where there's just a real deficit of public knowledge about the school board, about public health, about whatever public demands we might consider. And it's clear even as the news business is being hollowed out across the country, there's this new potential set of technological solutions which would be to create AIs that can vacuum up huge amounts of public data and create stories. And there are people experimenting in this way with an approach towards using AI to create essentially an online newspaper.
And so I was just trying to think through the risks and one is certainly that as such a model would do agenda setting and framing and narrative creation within a community. The community then would potentially respond in a certain way, and that might mean, let's show up in either an approving or an angry way at the school board meeting, and then the AI then processes that and then you can see very quickly how the AI's agenda setting could be influenced by these sort of feedback loops. And I don't pretend that news reporting has ever been perfect and there've been generations of researchers who have critiqued news media reporting for all of its human flaws. But if the AI researchers are genuine in saying that safety and alignment are the highest virtue in the model creation, we're going to have to figure out how if we allow the models to influence human behavior and communities by creating public knowledge, how do we make sure that it is aligned with human values and human preferences? And again, it's a philosophical point, but I think there is a danger there that we really haven't thought through.
That said, I do think AI can create tremendous efficiencies within newsrooms. For example, many reporters are parachute into a new beat, don't know much about what they're covering, and AI can surface all of the archives, for example, or all of the relevant reporting from the past. So we can imagine reporters being empowered by AI tools as well as potentially being disempowered.
Justin Hendrix:
Elise, one of the words you use in your piece is objectivity and this idea that Google, in terms of what it's trying to do, they vaunted this idea that they're providing facts and trying to provide a kind of objective set of information. I don't know, when I think about journalism and I think about the possibility of replacing journalism, I think a lot of folks would imagine that's one of the goals, is to improve the objectivity of the information that's being provided whether you believe in that term or not. But I don't know, I'll just leave it there with that prompt to you, objectivity and its problems.
Elise Silva:
Sure. I've been thinking about this for quite a while. My background is in university teaching and sometimes teaching rhetoric classes, and so thinking about how everything is contextual and that objectivity itself is and can be a very persuasive mechanism to gain trust and it oftentimes overshadows some of the less objective things that are happening in any communication and any type of communication and any type of information creation dissemination nexus. And so something I like to think about a lot is that nothing about information creation, information finding, or information usage is ever neutral. It can't be neutral. There will always be political dynamics to that, in terms of whose information is prioritized to be seen, whose information isn't, who has access to or is able to create information. So for example, we have huge deficits in Wikipedia editors and representation there, and so there's really nothing neutral about information ecosystems and while we want to trust and trust where these sources and we are drawn oftentimes towards objective tone neutrality for that, it's always important for us to remember these other things.
And I think, and I'm going to just shift here to respond to some of what I was hearing before about local journalism. Some of the work that we're doing right now at the University of Pittsburgh's disinformation lab is thinking about what local information ecosystems look like, and specifically within wider trends and a crisis of trust in anything, in government, in religion, in media, in media and in all of these types of places, and it turns out that trust is oftentimes earned from people. Right? It's earned on a micro level, it's earned with a journalist talking to people and uncovering the processes that journalists go through in order to vet information. It's on those really small levels, and so thinking that AI can solve this, I think sidesteps some of the wider very social realities that we're living right now and the politics of trust that we are experiencing.
Justin Hendrix:
One of the other things, John, that you get into in your paper is the idea of AI agents and the extent to which those will begin to overrun the internet. We'll probably interact with them in a variety of different contexts on social media, in search and maybe in various other domains as well. At least makes me think about, what does that do to our experience of receiving information, arguing, coming to know about certain things? And yet we know these AI agents, this is the main goal, right? We see DeepMind publishing papers about it, we see OpenAI and Anthropic essentially offering these products as a service. I don't know, how did these fit into your conceptual framework?
John Wihbey:
This is a bit futuristic, but obviously as you say, the research literature and all the noise from Silicon Valley seems to indicate that this is the next wave of the generative AI revolution, and there's I think a broad aspiration to create highly personalized agents that trained on our data like a proxy for Justin in the information environment who can do search and discovery, communication, buying, planning a trip, whatever it is, in a way that is consistent and aligned with your values and your interests, and there've been a bunch of tech leaders who have articulated that.
I think there's a lot to like about that model at one level, but how the agents would interact with one another and under what conditions, with what rules and with what constraints, is to me a really, really risky area that I think is going to require a lot of rules and regulations and a lot of careful thinking. And I don't think we are anywhere close to having, despite what Congress and the White House and other folks around the world are doing, we're just not close to having a real solid framework for guiding that future. And certainly, basic deliberative democracy functions about how we make everything from local to national decisions, are going to have to be structured in new ways as we empower these kind of proxies.
There are people who are talking about assemblies and other forms of democratic debate and deliberation. I think that's exciting, getting humans together in these sort of structured ways and those could then help guide the development of AI and the rules of the road for maybe agents, but this is a really uncertain future and I think it carries a lot of risk.
Justin Hendrix:
Elise, you talk about what you call seductive synthesis, a set of concerns including enfeeblement, possibly the impairment of moral decision-making processes. Are these agents likely, you think, to bring along those types of challenges?
Elise Silva:
I think that my biggest concern are people losing the ability to look at a variety of different sources, analyze those sources, and be able to put them into conversation with one another because that is a hard-won skill. It takes a really long time for somebody who's coming out of higher education and teaching and that type of stuff, we try very hard to help our students understand how to synthesize information, and if that's being done, it makes it seem so flat. We really lose, I think, the texture of different source types, we run the risk of homogenizing perspectives, arguments, and yeah, and I think that the way that it's synthesized for us really runs the risk of people being unable to do that themselves.
And I know that this, it echoes anxieties throughout history about tech and about people not being able to do things for themselves and we're okay still, but I do think that synthesis and especially when you're looking at information objects, being able to look at them in context with one another and not completely decontextualize as its own thing, is a really important understanding of how information is created and how you are an information creator as well, not just a consumer.
Justin Hendrix:
It feels like that's, I don't know, something I come back to in my head a lot, this fear of almost the information environment being smushed or flattened somehow, that's the best I can come up with in terms of the way I see it my head. I feel like we see early evidence of this that even right now in some of the actions that the social platforms are taking to push out political content or push out extremist rhetoric in certain cases or deamplify or not apply the recommender systems to that type of language, strikes me that's an even greater possibility when we start to introduce more of these technologies into the environment. And some of that mess that you're talking about, that sort of really just human artifacts of information get swept away or swept off into the corner.
Elise Silva:
Yeah, absolutely. There's a phenomenon known as container collapse, where our understanding of what information is by its shape, it's more traditional physical shape, is very much flattened when we're looking at it online. And so we're like, I used to be able to hold a book and I understand what this does as a book, but now I'm looking online. I'm like, what is this? Is this a blog? Is this coming from a journalist? And that's really hard to discern, and I think this is just the next step of a container collapse. We have even less context from which to understand what the information object is and therefore, how to evaluate it or assign it value to us as people who are making decisions, as people who are developing worldviews, as people who are conducting research and all sorts of types of things.
Justin Hendrix:
John, the part of your paper this makes me think about a little bit is the part that's about polling and the extent to which people are using LLM systems now, not just to provide information with regard to prompts, but to engage people in more extended conversations, asking open-ended questions, beginning to draw much more rich responses from individuals. We've even seen this in some social science. I've seen some interesting work recently on engaging people who have conspiracy theory beliefs and trying to suss out the limits of those beliefs and whether it's possible to change some of those beliefs by engaging with LLMs. I don't know, what do you make of the ability not only to I guess, provide information, but also to measure and at the same time shape public opinion?
John Wihbey:
The use of AI tools, and I've seen some of the same research I think at MIT, I do think AI tools for social science inquiry, that's a very intriguing pathway and bravo, I think we should explore it more. I think my concern was the use of LLMs to simulate human opinion on war, abortion, social policy, public policy, generally, and the potential misuse of that kind of data.
And some of the papers I reviewed, and I'm not a polling expert, I do some survey research, but the conclusion was that if the data is not well established in the model, LLMs have a hard time, first of all, anticipating unexpected events and how publics might respond. But second of all, breaking down the demographics of a potential set of respondents. So if you imagine almost a series of LLMs who are standing in for 1,000 representative Americans, what you would get, what if X or Y were to be proposed, how would they respond? The LLMs are pretty bad at it, at least so far, if there isn't really good training data that tracks very consistently with whatever issue you're presenting. And so my concern would just be that, I mean, polling is actually increasingly difficult because you can't reach people on phones and there's a whole representative samples are almost impossible to get nowadays. They're almost all these stratified, quota-based approaches.
But I just wonder if people start blending, for example, simulated LLM results with some human results and just trying to do weighting, but you may end up publicly releasing what purport to be polls that really actually don't represent what human beings think. Just as a provocation, we might consider what you'd think of as like the Barack Obama problem, was Barack Hussein Obama, a black American, a viable candidate in 2006? According to all past human training data, maybe not, right? And we could imagine the next, and it doesn't have to be a political point, it could be a conservative politician, but there are just ways in which human opinion takes interesting twists and turns that are just unpredictable, but an information environment that's heavily mediating based on past data and past preferences, I could imagine being deeply problematic for all sorts of reasons.
With the originalism movement in the conservative legal space, emerge, right? When all the LLMS say this is what conservatives think about constitutional doctrine, I have no idea. Can you come up with lots of different examples. Elise, I don't know what you think of that.
Elise Silva:
Yeah, I've been thinking a lot about this idea of mediation as it being this semi-translucent thing through which we experience the world that doesn't want to be seen, but we always have to realize it's there and that takes a lot. Right now it's taking a lot of effort on our part and that worries me, because before if you're thinking of an information environment, you're somewhat aware that these are coming from different sources and therefore different contexts, but now, instead of a place to browse, the internet is now a place to get a quick answer. It has been for a long time, but I think that there's a great power in shaping understandings of the world and opinions of the world, if you just have one voice through which that's happening that like I said, you have to always remember what it's doing because it seems so inevitable. It really isn't meant for you to linger on it, to think about the way that it's functioning as an intermediary and the way that it might be shaping how you think.
John Wihbey:
Yeah, and one of the things that really troubles me is that the confident tone of so much of what's produced by these generative models, it really purports in tone and in presentation to have this kind of human expert authority that actually it has not earned. And yes, it may be smarter than us at doing probability, but I worry about that simulation of human expertise being misleading.
Elise Silva:
Yeah, and I think that's an issue of objectivity as well, right? There's this constructed tone, this constructed sense that we can believe what's happening here. And authority is such a good word because even more than reliability or credibility, when you think of something authoritative, you think of something that really makes people do something or act, rather than maybe just believe something, that there's another layer to that.
Justin Hendrix:
I want to get to what both of you think we need to do at the moment. Both of you suggest as researchers always do, that we need to do more research. So I suppose we can put that one aside unless we want to get into what specific areas of research we need to do.
John, I find you almost calling for a sort of adoption of a set of norms about how we're going to approach these technologies and humility, if you will, or a kind of acceptance of their fallibility and all of the challenges they may create, but I find that very much at odds with what's actually going on in the world at the moment.
John Wihbey:
Yeah, I find it at odds with the world as well. I think the impulse with LLMs, at least in this first iteration of public-facing interfaces has been this question-and-answer paradigm. Humans have queries and the machine should be able to give definitive, excellent answers. What I worry about is, Elise and I just talked about authority, but what I worry is that we don't have a conception of what is the opposite, what is modesty? What does epistemic modesty look like? What is the machine technology saying? I have limited understanding and so I cannot give you a definitive, and this isn't just on basic factual questions, but questions about what people should do, how they should behave, personal health, education choices, all these different things. How do we make sure that the machines identify themselves as agents and also as agents with limited capability, even if they can purport to have vast capability?
To put this really concretely, think about these attempts to create AI-simulated news anchors that are just up there saying things, and I think that's where in the context of journalism, we really get into trouble, right? Where the AI actually just attempts to be a journalist, which I think is just the wrong approach, and there's this idea within the literature of human-centered AI, which I like a lot, and the anthropomorphic vision of the AI as just a human only better, I think is really problematic and what we need to think about is how can that AI be representing itself in knowledge space, human space as something other than human, but something that helps humans in an aligned way? And I don't know what that means concretely, but I think that's where I'm headed intellectually.
Elise Silva:
I have so many thoughts right now and my first impulse is to think about what users can do because I don't have a whole lot of faith in big tech. And thinking about what types of information literacy interventions we can be making, especially for young people who are going to be growing up using this technology, it's going to be really shaping their lived experiences and I think that type of instruction or those types of interventions might look like focusing on that which is invisible, rather than just what is visible. Like I said, the platform, what's happening behind the scenes.
I also think it's a focus on process, so the processes by which information is created, the processes by which information is accessed and the different forms that takes in evolving information environments, but I think on, that puts the onus on the user and I don't think that's fair. I think we need to be demanding a lot more accountability from these companies that are promising that this is going to supercharge our, that word is everywhere, supercharge our research. And research, there are some information needs that require a very quick answer, but there are a lot of them that don't need to be supercharged, that need to slow down, maybe not super slow but slower, and somehow require or create an accountability mechanism where these interfaces are encouraging exploratory behaviors, encouraging people to ask questions that the model itself isn't the only one that's producing, because if you've used the new AI overviews, it's like prompting you with follow up questions. That gives the user a little bit more autonomy as they're interacting with it to be a human and to direct it rather than be directed by it. And I think that that's an accountability that we need to be creating for these companies.
Justin Hendrix:
Lawmakers, policymakers, there may be one or two listening to this podcast. If you are in the room with them at the moment, sitting at the hearing at the dais or maybe in one of these inside forums that are held behind closed doors, what would you want to tell lawmakers? I can start with you Elise, if you'd like.
Elise Silva:
Incentivize slower deployment somehow. There is such a race to be the next big thing, and Google specifically because that's what I talked about in Vine, 90% of the market share, everybody's going to be using this technology. We have to incentivize slower deployments of these technologies.
Justin Hendrix:
It did occur to me that at the moment that some of the AI search, AI overview kind of nonsense was rolling over social media, people posting lots of bizarre examples of searches. A lot of folks, and I was one of them, were also mourning the fact that all this is happening in the midst of so many attacks on public libraries, for instance, and other kind of institutions where we try to help people understand the world or find information maybe in a more slow and humane way.
Elise Silva:
Yeah, and reminding people somehow that these information access hubs exist aside from Google is its own difficulty, but yeah.
Justin Hendrix:
What about you, John? If you got the invitation to sit in the committee.
John Wihbey:
I think Elise is right, the incentives are everything. I guess, I would put a lot more legal liability on the companies that are producing LLMs, maybe not in an extreme way, but more so than we have, for example, with the social media companies with Section 230. And I do think as these become speech generating or communication generating platforms, we have to rethink whether section 230 is really the right paradigm to evaluate what they might be legally responsible for because it just seems a different order, it just seems like we're dealing with something quite different now. We're not merely dealing with user generated content, but content that is generated by models that are constructed by the employees of the companies, and so that seems to me categorically different.
Now, it may be that we distinguish between personalized LLMs that are like ChatGPT that are just talking to you and AI overview results, which are effectively public knowledge for internet users with the same kinds of queries. So we're just going to need a lot of careful thinking, and I do think we'll probably need some kind of legislation to grapple with what happens when an LLM encourages a bunch of young people to take blah, blah, blah substance in order to cure some condition and they do. What's the liability framework there? And across all human experience, there are going to be different variations of that.
I also think on the IP and copyright issues, we're going to need to update all of those laws because I do think creators, and that would include not just musicians and artists, but also journalists and other folks who are not highly paid professionals across the society, very vulnerable to shocks within the economy. We're going to need to be able to protect them because they're producing in some ways the most important information and knowledge and cultural products that we have as humans. I would seek to protect them as well.
Justin Hendrix:
Both of you end your pieces with essentially calls to be slow, have meaningful conversations, watch these issues very closely and give them great care.
John, you say, "The danger of epistemic risk presents a profound problem in the coming AI era, one requiring constant attention to issues of public knowledge and human autonomy within democratic systems." Let me just ask you a final question, each of you. Long run optimism, pessimism about where AI is taking us with regards to democracy having considered these things and looked at this trajectory. Can you see a point in the future where we've got beyond the current moment, which seems almost like a cartoon, to a place where these systems are actually serving us and helping us build a better democracy?
John Wihbey:
With all epistemic modesty, I think the future very much depends on the choices we make in the next few years. One of the things I do in the paper is just go through some of the concerns about the information environment in past generations. At one point it was about mass media and propaganda. You think of Chomsky. In recent times, it's been about algorithms and social media and the way that it's filtered, experience and created filter bubbles around information. All of those fears I think resulted in lots of harm, but then humans figured out a way to muddle through and come out on the other side as a species still relatively intact. I suspect that's probably what will happen here as well, as the harms and the extremes get some kind of either public policy attention or collective action happens, but there's going to be a push and pull and I think we should be ready to advocate very strongly for protections and constraints, even as I do think the AI technologies could contribute to democratic debate and some other areas and could certainly be helpful in knowledge discovery, but I think we have to just be really careful.
Elise Silva:
I agree. I think that looking at history is really helpful here. There were huge concerns and anxieties about radio and about television and the ways that would affect how people got news and we went on, and people have been creative and resilient and use those technologies. What I'm going to say is that oftentimes these types of technologies, and I think generative AI specifically, is positioned or it is advertised as a fix to things, right? This can fix the hard stuff, tech can be the thing that fixes it, but tech is never going to be able to be the only thing that fixes sociotechnical society. Sometimes it causes problems that then it itself cannot fix, this becomes a wider issue.
And so if there's a bright future, if there's a positive future out there, it's going to require us to work with it. It's going to require people to have autonomy and think about it as a thought partner, and I think that's going to be really important going forward, is realizing that we're using this tech within a social system. That's how we solve problems, like the tech itself can't.
Justin Hendrix:
I'm grateful for the two of you for taking the time to speak to me about this and I look forward to carrying on the conversation on Tech Policy Press and elsewhere when you publish additional papers. I think we're going to be working on this one for a while.
John Wihbey:
Thank you.
Elise Silva:
Appreciate it.
Authors
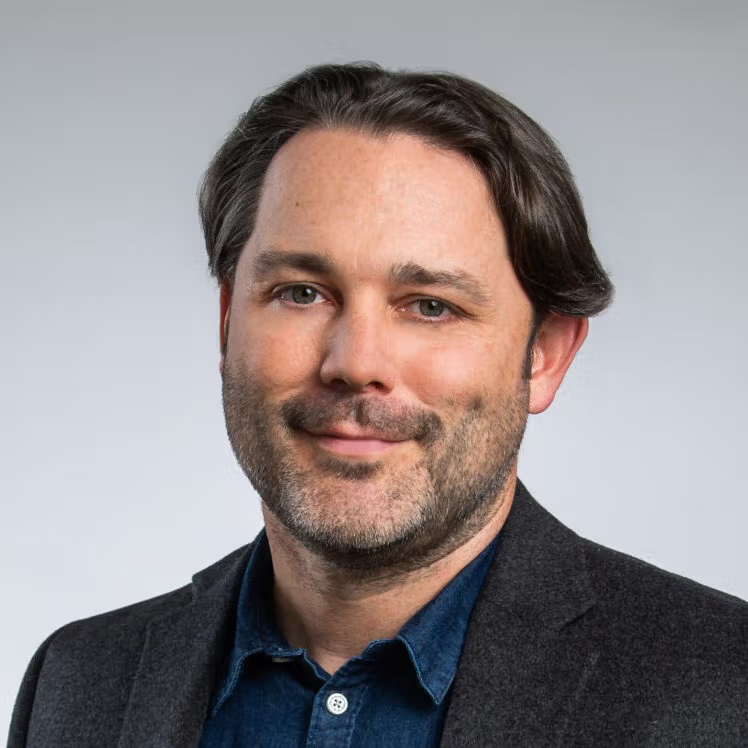